논문 요약
1. Paper Bibliography
논문 제목
- Revisiting temporal modeling for video super-resolution
저자
- Isobe et al.
출판 정보 / 학술대회 발표 정보
- arXiv preprint arXiv:2008.05765 (2020)
년도
- 2020
2. Problems & Motivations
논문에서 언급된 현 VSR 연구들에서의 문제점 정리 + 관련 연구
Previous VSR works
1) Explicit Motion Compensation based methods [1, 13, 20, 23, 16]
- 정확하지 않은 ME, alignment가 결과에 악영향을 줄 수 있다
- optical flow를 계산하는 것은 컴퓨팅 비용이 비싸고 실시간으로 할 수 없게 된다
2) Implicit Motion Compensation based methods [4, 8, 12, 16, 25, 27]
Temporal modeling framework
1) 2D with early fusion:
2) 3D with slow fusion:
3) Recurrent Neural Network (RNN):
Temporal modeling approaches를 바로 비교하기 어렵다
- different training sets and loss functions
- different network depth
[1] Jose Caballero, Christian Ledig, Andrew Aitken, Alejandro Acosta, Johannes Totz, Zehan Wang, and Wenzhe Shi. Real-time video super-resolution with spatio-temporal networks and motion compensation. In CVPR, 2017.
[4] Dario Fuoli, Shuhang Gu, and Radu Timofte. Efficient video super-resolution through recurrent latent space propagation. CoRR, abs/1909.08080, 2019.
[8] Muhammad Haris, Gregory Shakhnarovich, and Norimichi Ukita. Recurrent backprojection network for video super-resolution. In CVPR, 2019.
[9] Yan Huang, Wei Wang, and Liang Wang. Bidirectional recurrent convolutional networks for multi-frame super-resolution. In NeurIPS, 2015.
[10] Takashi Isobe, Xu Jia, Shuhang Gu, Songjiang Li, Shengjin Wang, and Qi Tian. Video super-resolution with recurrent structure-detail network. 2020.
[11] Takashi Isobe, Songjiang Li, Xu Jia, Shanxin Yuan, Gregory Slabaugh, Chunjing Xu, Ya-Li Li, Shengjin Wang, and Qi Tian. Video super-resolution with temporal group attention. In CVPR, 2020.
[12] Younghyun Jo, Seoung Wug Oh, Jaeyeon Kang, and Seon Joo Kim. Deep video superresolution network using dynamic upsampling filters without explicit motion compensation. In CVPR, 2018.
[13] Armin Kappeler, Seunghwan Yoo, Qiqin Dai, and Aggelos K Katsaggelos. Video super-resolution with convolutional neural networks. IEEE Transactions on Computational Imaging, 2(2):109–122, 2016.
[16] Soo Ye Kim, Jeongyeon Lim, Taeyoung Na, and Munchurl Kim. 3dsrnet: Video superresolution using 3d convolutional neural networks. CoRR, abs/1812.09079, 2018.
[20] Mehdi SM Sajjadi, Raviteja Vemulapalli, and Matthew Brown. Frame-recurrent video super-resolution. In CVPR, 2018.
[23] Xin Tao, Hongyun Gao, Renjie Liao, Jue Wang, and Jiaya Jia. Detail-revealing deep video super-resolution. In ICCV, 2017.
[24] Yapeng Tian, Yulun Zhang, Yun Fu, and Chenliang Xu. Tdan: Temporally deformable alignment network for video super-resolution. CoRR, abs/1812.02898, 2018.
[25] Xintao Wang, Kelvin CK Chan, Ke Yu, Chao Dong, and Chen Change Loy. Edvr: Video restoration with enhanced deformable convolutional networks. In CVPR Workshops, 2019.
[26] Tianfan Xue, Baian Chen, Jiajun Wu, Donglai Wei, and William T Freeman. Video enhancement with task-oriented flow. International Journal of Computer Vision, 127 (8):1106–1125, 2019.
[27] Peng Yi, Zhongyuan Wang, Kui Jiang, Junjun Jiang, and Jiayi Ma. Progressive fusion video super-resolution network via exploiting non-local spatio-temporal correlations. In ICCV, 2019.
3. Proposed Solutions
논문에서 제안하는 해결책들 정리
Network Design
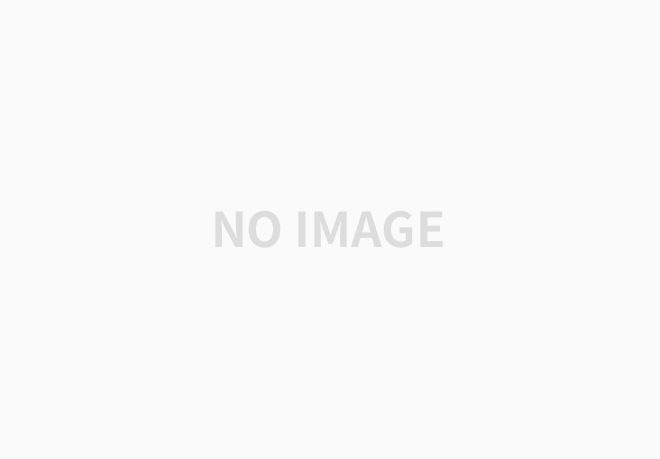
1) 2D CNN with early fusion
- EDSR[18]을 따라 여러 residual blocks를 이용한 2D CNN 디자인: 각 블록은 3x3 convolution layer + ReLU 구성이다
- 2T+1 연속된 frames를 입력으로 받고 concat된다 (크기 NC x H x W)
- 만들어진 residual map의 크기는 H x W x Cr^2이며 depth-to-space 연산으로 얻는다
- 최종 HR이미지는 얻은 residual map과 LR을 bicubic으로 upsample한 이미지를 합쳐서 얻는다
2) 3D CNN with slow fusion
- 2D residual blocks의 2D convolutional layers를 3x3x3 convolutional layers로 수정
- 정당한 비교를 위해 2D와 3D의 네트워크 깊이를 같게함
- 3차원 필터가 temporal axis와 spatial axis로 움직이며 sptatial-temporal한 정보를 얻는다
- 보통 3D filter의 temporal dimension depth은 input sequence보다 작은 경우가 많다
- 입력 텐서의 크기는 C x N x H x W이다
- 프레임 수 감소를 방지하기 위해 0으로 채워진 frame 2개를 temporal axis에 추가한다
3) RNN
- time step t에서 hidden state는 1) previous output Ot-1, 2) previous hidden state features ht-1, 3) two adjacent frames I{t-1,t}를 입력으로 받는다
- 고주파 텍스처 디테일은 이전 레이어로부터 보충 정보를 얻는다
- VSR에서 RNN은 gradient vanishing 문제를 많이 겪는다
- 이를 해결하기 위해 Residual Recurrent Network (RRN)은 identity skip connection을 사용해 layers간 residual mapping을 했다
- 이러한 디자인은 자연스러운 정보 흐름을 주며 텍스처 정보를 오랜 기간 남아있게 하여 RNN이 더 긴 시퀀스를 쉽게 처리하게하고 gradient vanishing 문제를 줄여준다


[18] Bee Lim, Sanghyun Son, Heewon Kim, Seungjun Nah, and Kyoung Mu Lee. Enhanced deep residual networks for single image super-resolution. In CVPR Workshops, 2017.
4. 입력의 형태
- LR patches 64x64 (HR frame에 가우시안 블러 1.6 적용)
5. 시간적 정보 모델링 프레임워크
기본 프레임워크 (2D CNN, 3D CNN, RNN, etc)
- RNN
구조에 기여한 바가 있다면?
- RNN과정 중 identity skip connection을 사용해 layers간 residual mapping을 해서 gradient vanishing 문제를 방지하고 연속성을 강화시켰다
6. 프레임 정렬 방식
Implicit (암시적) or Explicit (명시적)
- implicit
추가 설명
-
7. 업샘플링 방식
- RRN을 통해 얻은 결과 + bicubic upsampled target frame (elemental-wise addition)
8. 그 외
모델 파라미터 개수
- RRN-S: 108M
- RRN-L: 193M
학습 데이터
Vimeo-90k
- 90k 7-video clips with various motions and diverse scenes
테스트 데이터
Vid4
- 4 scenes with various motion and occlusion
SPMCS, UDM10
- diverse scenes with considerable high-resolution frames than Vid4

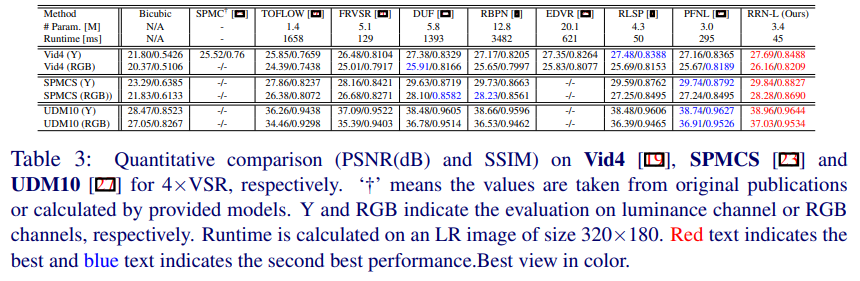
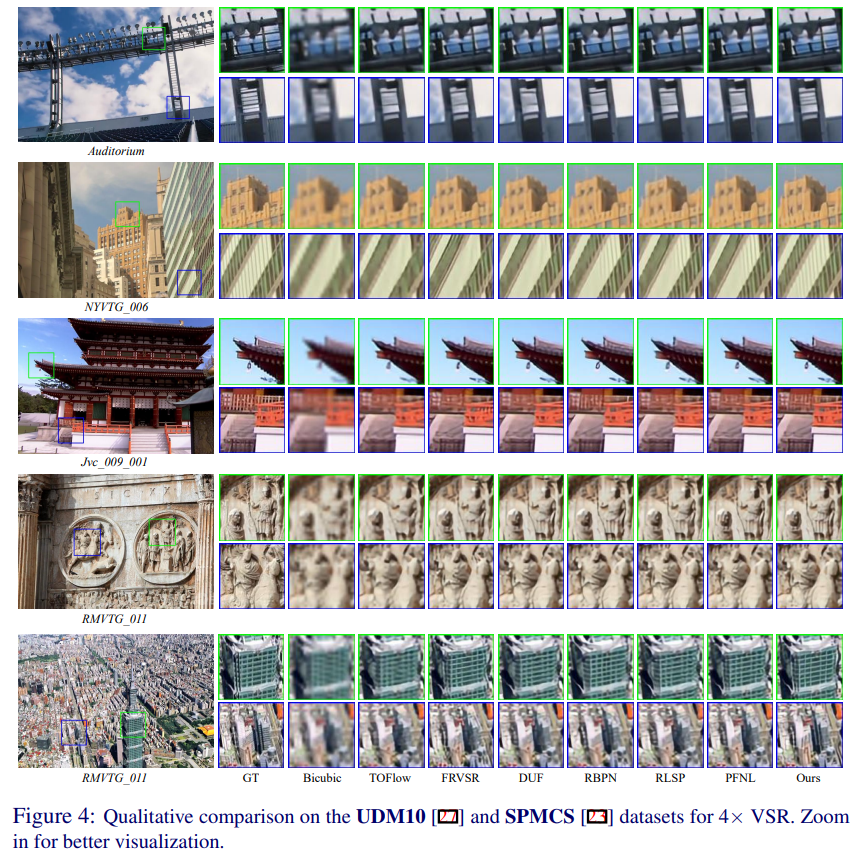
논문 분석
1. 앞서 정리한 논문들에 대한 비평들 중 해당 논문에서 해결된 바가 있다면 정리
-
2. 해당 논문에 대한 비평(Critique)
1)
2)
3)
Google Scholar Link
https://scholar.google.co.kr/scholar?hl=ko&as_sdt=0%2C5&q=revisiting+temporal+modeling&oq=
Google 학술 검색
검색결과 약 220,000개 (0.03초)
scholar.google.co.kr
GitHub
https://github.com/junpan19/RRN
GitHub - junpan19/RRN
Contribute to junpan19/RRN development by creating an account on GitHub.
github.com
댓글